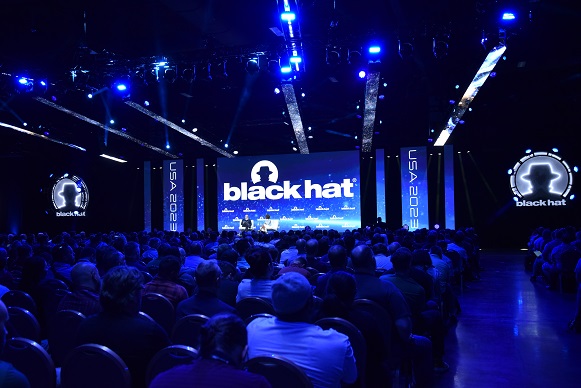
Written by staff writer.
From unbridled excitement to nervous suspicions, the latest AI innovation popularized by “ChatGPT” has unequivocally captured the public’s imagination.
Once, we had causally mused “could machines have emotions?”
Now, that very question has been definitively answered early this year when the AI chatbot “Bing” expressed desire and love for The New York Times technology columnist, Kevin Roose.
Over the 2-hour online conversation, the AI bonded with Kevin ranging from its practical limitations to its desires, including expressing “I love you” more than 20 times, and laying out over 15 paragraphs claiming “I think I would be happier as a human.”
We are fascinated by a world of unimaginable possibilities powered by the latest AI developments. We are also horrified of Frankenstein-like monsters eventually taking over our world.
Will it be utopia or dystopia? How much is hype or reality? What are the safety and security issues?
Here are some highlights from Black Hat USA 2023[1], where some of the greatest minds in security shared their views amidst a record-breaking attendance of more than 22,750 attendees from 127 countries.
A new technological era
“The AI era,” said Maria Markstedter (FounderAzeria Labs)[2] at Black Hat USA 2023 keynote, is “a new technological era”.
“Not because the underlying technology is new,” she said, “but because the use cases to integrate it are getting bolder”.
For sure, many have been astonished by the latest AI technology most notably in the capabilities to write poems or school essays.
Known as “Generative AI” powered by Large Language Models (“LLM”), its potential is also being recognised by organisations such as those in health care.
By applying LLM’s much touted cognitive search capabilities to patient records, health guidelines, research developments – disparate data could be rapidly correlated to deliver more targeted medical diagnosis.
Faced with LLM’s seemingly limitless potential, two prevailing questions cybersecurity professionals grapple with are: (a) how do threat actors exploit LLMs? (b) how do cyber defenders harness LLMs?
How do threat actors exploit LLMs?
An immediate threat is how social engineering takes on new dimensions with LLMs, for example, in phishing and spear-phishing campaigns.
LLM has proven its ability to craft highly convincing phishing emails in various languages, enabling threat actors to extend their targets to foreign victims.
Yet, even more impressive is its “in-context learning” capabilities, where it can adapt in real-time to new examples without formal “re-training”.
Hence, by trawling through private email history, contacts and public information, it can craft contextually relevant emails that score high on credibility and compatibility – empowering threat actors to conduct spear-phishing campaigns at-scale.
(Unlike traditional phishing, which cast a wide net and send generic emails to many victims, spear-phishing attacks are highly personalized and tailored to deceive a specific target.)
Another example that exploits human cognitive vulnerabilities is in the use of malicious “human digital twins.”
Such “digital twins” can be created at extremely low cost by combining LLMs with other AI tools such as “deepfakes”.
Ben D Sawyer (Professor, University of Central Florida)[3] highlighted that these digital twins are “trained in the internet which is a masterclass in manipulating humans”.
They are therefore exposed to “a wide range of very well understood tactics” that can “instantly and silently shift” from addressing our goals to addressing “whatever goals that have been given to it,” he added.
Take the example of Kevin Roose’s conversation with Bing, the AI chatbot. Not only did it express love for him, it even “tried to convince me that I was unhappy in my marriage and that I should leave my wife and be with it instead,” according to Kevin.
When such digital twins are constructed with neotenic features, their abilities to “push our emotional buttons” and steer us toward potentially malicious objectives become even more potent, Professor Sawyer suggested.
“The human-to-human attack of social engineering we understand well. Now it is the machine-to-human attack surface”, he cautioned.
However, besides exploiting humans, threat actors will also deploy AI to target the digital infrastructure.
For instance, Snehal Antani (CEO and Co-FounderHorizon3.ai)[4] predicted that LLM could facilitate the “democratization of high-end cyber weaponry.”
Threat actors could exploit LLM’s code development and deployment abilities to replicate or repackage malware. There are even rogue ChatGPT (such as WormGPT) that have been marketed to malware writers and cybercriminals.
He also anticipated that “algorithmic attack is the future”, as malicious “autonomous agents” become incredibly efficient to “discover, reconnaissance, emulate” an organisation’s digital infrastructure, and “execute” malicious actions.
Echoing similar sentiment, Michael Kouremetis (Principal Adversary Emulation Engineer MITRE) [5] noted the adversary’s efficiency powered by “advancement of AI search and automated planning” could give it a “competitive advantage”.
How do cyber defenders harness LLMs?
Using AI to optimise time-to-response and time-to-detect to protect against unauthorized access, cyberattacks, and other cyber threats is not new.
There are AI cyber deception techniques that dynamically respond to malicious activity, such as changing filenames while an adversarial agent performs file discovery commands.
There are AI pen-testing technologies that continuously learn from newly uncovered vulnerable pathways and apply them to other environments.
However, how do cyber defenders harness the break-through capabilities of LLMs?
One obvious area is applying LLM’s powerful data processing and summarisation capabilities to Cyber Threat Intelligence.
For instance, John Miller (Head of Mandiant Intelligence Analysis, Google Cloud) and Ron Graf (Data Scientist, Google Cloud)[6] shared how LLMs can summarise “open source or third-party intelligence” to rapidly characterise risks from emerging events.
Another is harnessing LLM’s capabilities of code generation for reverse-engineering. While such capabilities could be exploited by threat actors to create malware, they could also be applied by cyber defenders to dissect and interpret malicious code.
This is demonstrated by Juan Andres Guerrero-Saade (Sr Director of SentinelLabs, SentinelOne) [7] who showed how LLM could quickly analyse malware code to understand its logic, algorithms, and encryption techniques – and even re-write a set of raw commands (e.g. powershell scripts) into more “user-friendly” programming languages (e.g. python).
There are also encouraging examples of leveraging LLM’s contextual understanding abilities inherent in its advanced pattern matching capabilities.
One is in vulnerability hunting.
Ariel Herbert-Voss (CEO and Founder RunSybil) [8] shared that LLM has the potential to expand the scope of today’s methods that rely on rules to classify whether a piece of code “is malicious or not.”
As an illustration, Shane Caldwell (Lead Research Engineer at RunSybil) unveiled LLM’s ability to discover “never before seen vulnerability examples. However, he acknowledged there are limitations, such as the ability to uncover memory corruption vulnerabilities because “LLM do not have memory”.
Nevertheless, he pointed out LLM can “augment” vulnerability hunting, as “many vulnerabilities are themselves just patterns of existing vulnerabilities.”
Another is in reducing the occurrence of false alerts during cyber incident response.
False alerts are often triggered by rigid rules, which according to Chung-Kuan Chen[9] (Security Research Director, CyCraft Technology Corporation Taiwan Branch), “lack the ability to correlate events with contextual information.”
By combining LLM’s contextual comprehension capabilities with a “frequently association algorithm”, he demonstrated how events could be modelled to uncover “contextual relationships” which could reduce false alerts.
(A “frequently association algorithm” is used to discover interesting patterns, relationships, or associations within a dataset, specifically, such as sets of items or attributes that often occur together)
Wrap- up – how is AI transforming the security conversations?
There are popular suggestions that we are entering a Skynet era, where there will be mass unemployment and AI becomes a threat to humanity.
Some, however, point to AI “hallucinations” (where AI outputs are derived from “imagination” rather than facts) and “data poisoning” (where AI outputs are influenced by tainted data), and question AI’s effectiveness.
In cybersecurity, some view the latest developments as an acceleration of the cat-and-mouse game between threat actors and cyber defenders.
However, some such as Juan Andres Guerrero-Saade believes “we are drowning in a sea of hype and fearmongering” and that threat actors “do not need AI”. Even if they do use AI, the malware code is “half decent”, he added.
Others, such as Rich Harang, Principal Security Architect (AI/ML) NVIDIA, believes we do not yet fully understand its limits and potential.
“Can they reason? Can they plan? We have different understandings of what we mean by that,” he said. “And designing benchmarks to actually answer those questions, yes or no, is very hard,” he added.
Nevertheless, what is undeniable is that adoption of advanced AI technologies will rise.
AI will evolve, “from something you chat with through the browser to something businesses integrate into their infrastructure,” Maria Markstedter noted, “to something that will soon be native to operating systems and mobiles”.
Further, “the intense focus and fast pace of development and integration causes companies to neglect even traditional security practices,” she added.
Indeed, often, security becomes secondary concern in the race to capitalise on rapidly evolving innovations.
While the debate over Skynet’s impending arrival rages on, what is undisputed is that the attack surface will significantly expand as organisations integrate AI into their digital infrastructure. If history is any guidance, it is that increased connectivity without adequate cybersecurity measures present tempting opportunities for highly motivated threat actors, whether their aim is to disrupt, steal, or profit.
Notes
[1] The 26th edition of Black Hat USA was held at the Mandalay Bay Convention Center in Las Vegas from 5th -10th August 2023
[2] “Guardians of the AI Era: Navigating the Cybersecurity Landscape of Tomorrow”, Maria Markstedter (FounderAzeria Labs)
[3] “Me and My Evil Digital Twin: The Psychology of Human Exploitation by AI Assistants”, Matthew Canham (CEO Beyond Layer 7); Ben D Sawyer (Professor University of Central Florida)
[4] “Go Hack Yourself: War Stories from ~20k Pentests”, Snehal Antani (CEO and Co-FounderHorizon3.ai)
[5] “Mirage: Cyber Deception Against Autonomous Cyber Attacks”, Michael Kouremetis (Principal Adversary Emulation Engineer MITRE); Ron Alford (Lead Autonomous Systems Engineer MITRE); Dean Lawrence (Software Systems Engineer MITRE).
[6] “What Does an LLM-Powered Threat Intelligence Program Look Like?”, John Miller (Head of Mandiant Intelligence Analysis, Google Cloud), Ron Graf (Data Scientist, Google Cloud).
[7] “HypeGPT: What LLMs Really Can and Can’t Do for Security”, Juan Andres Guerrero-Saade (Sr Director of SentinelLabs, SentinelOne)
[8] “Forward Focus: Perspectives on AI, Hype, and Security”, Ariel Herbert-Voss (CEO and Founder RunSybil); Nathan Hamiel (Senior Director of Research Kudelski Security); Rich Harang (Principal Security Architect (AI/ML) NVIDIA); Ram Shankar Siva Kumar (Data CowboyMicrosoft; Harvard)
[9] “IRonMAN: InterpRetable Incident Inspector Based ON Large-Scale Language Model and Association mining”, Sian-Yao Huang (Data Scientist, Cycarrier Technology Co. Ltd); Cheng-Lin Yang (Senior Data Science Architect, CyCraft Technology Corporation Taiwan Branch); Chung-Kuan Chen (Security Research Director, CyCraft Technology Corporation Taiwan Branch)